How Industrial DataOps Improves Data Security & Compliance
Discover how Industrial DataOps strengthens data security in cPdM through encrypted communication, real-time monitoring, and robust governance protocols.
share this

1.0. Introduction: Strengthening Data Security and Compliance with Industrial DataOps
In today’s industrial landscape, ensuring data security and maintaining regulatory compliance is more critical than ever. Industrial DataOps offers a strategic framework that combines cutting-edge technologies with robust management practices to protect sensitive information and meet stringent industry regulations.
By implementing secure data flows, real-time monitoring systems, and advanced governance protocols, organizations can effectively safeguard their operational data while adhering to regulatory standards. This dual approach not only reduces security risks but also enhances compliance readiness, boosting overall cyber resilience.
As industries undergo digital transformation, the importance of Industrial DataOps will continue to grow. With increasing connectivity, new technologies, and evolving regulations, adopting a proactive data management strategy will be essential. This ensures that organizations not only protect sensitive data but also build trust with stakeholders by demonstrating a clear commitment to security and compliance.
2.0. Data Security Mechanisms: Safeguarding Industrial Information with Industrial DataOps
In the industrial sector, protecting sensitive data is paramount. Industrial DataOps offers a comprehensive framework that integrates secure data flows, real-time monitoring, and robust access controls to prevent unauthorized access and ensure compliance with regulatory standards.

2.1. Secure Data Flow Management
Efficiently managing data flows across industrial systems—including sensors, machines, and enterprise applications—is a core principle of Industrial DataOps. This involves:
- Data Access Control:
- Implementing Role-Based Access Control (RBAC) ensures that only authorized personnel can access sensitive data.
- This minimizes the risk of insider threats and accidental data exposure.
- Continuous Data Flow Monitoring:
- Real-time monitoring systems detect unauthorized access or anomalies.
- Organizations can set up alerts for unusual patterns, enabling swift responses to potential breaches.
2.2. Use of Secure Communication Protocols
To maintain data integrity during transmission, Industrial DataOps uses secure communication protocols specifically designed for industrial environments.
- Encryption Standards:
- Protocols such as TLS (Transport Layer Security) and SSL (Secure Sockets Layer) encrypt data in transit.
- This ensures that intercepted data is unreadable to unauthorized users.
- Secure Messaging Protocols:
- MQTT (Message Queuing Telemetry Transport) and OPC UA (Open Platform Communications Unified Architecture) provide secure, low-latency communication between devices.
- These protocols are widely adopted in industrial IoT networks for their reliability and security.
2.3. Contextualization and Standardization of Data
Standardizing and contextualizing data enhances both security and operational efficiency by reducing vulnerabilities linked to inconsistent data formats.
- Data Normalization:
- Converting data from various sources into a common format reduces inconsistencies and errors.
- This minimizes security gaps caused by incompatible or corrupted data.
- Metadata Management:
- Adding metadata tags provides valuable context, such as data lineage, sensitivity levels, and usage rights.
- This improves data traceability and reinforces security.
2.4. Edge Computing for Local Processing
Leveraging edge computing enhances data security by processing information closer to the source rather than sending it to external servers.
- Reduced Latency:
- Local processing reduces response times for critical operations, such as predictive maintenance and quality control.
- This ensures real-time decision-making without the risks associated with remote data transfers.
- Minimized Data Transmission:
- Less data is sent over networks, lowering the risk of interception or breaches during transmission.
- This reduces the attack surface for potential cyber threats.
2.5. Real-Time Monitoring and Alerts
Effective real-time monitoring is essential for identifying and mitigating security threats as they occur.
- Anomaly Detection Systems:
- Machine learning algorithms analyze data patterns to detect anomalies, such as suspicious access attempts or unexpected data spikes.
- This helps organizations identify potential threats early.
- Incident Response Integration:
- Automated alerts trigger predefined incident response protocols.
- This ensures swift action, reducing the impact of security incidents.
2.6. Intellectual Property Protection
In industrial sectors, safeguarding proprietary information is crucial for maintaining competitive advantage.
- Data Masking Techniques:
- Sensitive information is masked or anonymized during analysis.
- This prevents unnecessary exposure when sharing data with third parties.
- Access Audits:
- Regular data access audits track who interacts with sensitive information.
- This ensures compliance with internal policies and helps organizations detect unauthorized activities.
3.0. Compliance with Regulatory Standards: Ensuring Data Security and Legal Adherence
In today’s industrial landscape, maintaining regulatory compliance is critical for safeguarding sensitive data and avoiding legal repercussions. Industrial DataOps streamlines compliance efforts by enforcing data governance policies, conducting regular risk assessments, and adhering to industry standards. This structured approach ensures that organizations meet regulatory requirements while enhancing data security and operational integrity.

3.1. Data Governance Policies
Establishing robust governance policies is foundational for compliance:
- Policy Frameworks: Organizations should develop comprehensive policies governing data management practices, including retention schedules, access controls, and incident reporting procedures.
- Training Programs: Regular training for employees on governance policies ensures that everyone understands their roles in maintaining compliance and security.
3.2. Data Classification and Risk Assessment
Classifying data based on its sensitivity helps organizations implement appropriate security measures:
- Data Classification Schemes: Implementing classification schemes (e.g., public, internal, confidential) allows organizations to prioritize resources effectively based on the level of risk associated with each category.
- Regular Risk Assessments: Conducting periodic risk assessments helps identify vulnerabilities within the system and informs necessary adjustments to security measures.
3.3. Adherence to Industry Standards
Compliance with established frameworks is essential for maintaining high-security standards:
- Framework Implementation: Utilizing frameworks such as the NIST Cybersecurity Framework or ISO 27001 provides guidelines for identifying risks, implementing controls, and continuously monitoring effectiveness.
- Third-Party Audits: Engaging external auditors to assess compliance with industry standards can provide an objective evaluation of an organization’s security posture.
3.4. Incident Response Programs
Having a well-defined incident response plan is critical for effective security management:
- Incident Response Team (IRT): Establishing a dedicated team responsible for managing incidents ensures that there are trained personnel ready to respond quickly.
- Post-Incident Reviews: After an incident occurs, conducting thorough reviews helps identify root causes and improve future response strategies.
4.0. Data Security and Compliance in Continuous Predictive Maintenance (cPdM)
The integration of Industrial DataOps into Continuous Predictive Maintenance (cPdM) significantly enhances data security and ensures regulatory compliance. By implementing structured data management practices, organizations can:
- Protect sensitive operational data.
- Improve predictive analytics accuracy.
- Strengthen compliance with industry regulations.

Key Mechanisms for Secure cPdM Implementation:
- Secure Data Acquisition: Encrypting sensor data using secure communication protocols.
- Access Control: Enforcing RBAC (Role-Based Access Control) to limit data access.
- Data Normalization: Standardizing sensor inputs to enhance analytics accuracy.
- Real-Time Monitoring: Using machine learning to detect anomalies and threats.
- Edge Computing: Processing data locally to reduce exposure to cyber risks.
By adopting these data security strategies, organizations can safeguard sensitive information, enhance operational integrity, and optimize predictive maintenance through proactive decision-making.
4.1. Data Security in cPdM
To ensure data security in cPdM systems, Industrial DataOps leverages advanced security protocols and real-time monitoring mechanisms that safeguard data at every stage from acquisition to analysis.
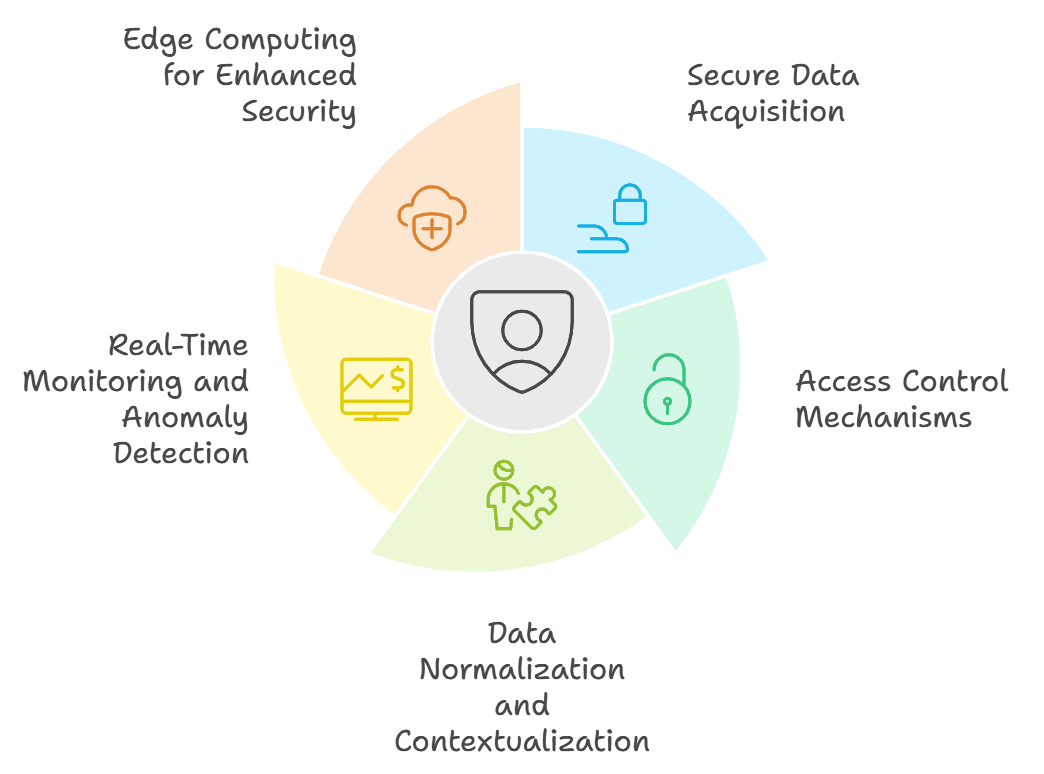
4.1.1. Secure Data Acquisition:
In cPdM, data is gathered from multiple sources, including:
- Vibration sensors (Piezo accelerometers).
- Temperature sensors (RTDs and thermocouples).
- Sound detectors (ultrasonic microphones).
To protect data integrity during acquisition, Industrial DataOps uses:
- Encrypted Communication Protocols:
- MQTT (Message Queuing Telemetry Transport) and OPC UA (Open Platform Communications Unified Architecture) secure data in transit.
- These protocols encrypt sensor data, preventing unauthorized interception and ensuring data integrity.
- Data Validation:
- Automated validation routines verify the authenticity and accuracy of incoming data, preventing manipulated or corrupted data from entering the system.
4.1.2. Access Control Mechanisms:
Robust access control is essential in cPdM to prevent unauthorized access to sensitive data.
- Role-Based Access Control (RBAC):
- Defines user roles with specific permissions (e.g., maintenance engineers, data scientists, managers).
- Ensures only authorized personnel can access critical maintenance data, reducing the risk of insider threats.
- Centralized Data Archives:
- Maintaining a consolidated archive of historical maintenance data helps detect patterns in equipment performance.
- This enables secure and accurate data analysis while restricting access to authorized personnel only.
4.1.3. Data Normalization and Contextualization:
To enhance data consistency and reliability for predictive analytics, Industrial DataOps standardizes and contextualizes sensor data.
- Data Normalization:
- Converts heterogeneous data formats into a standardized structure.
- For example:
- Temperature readings from RTDs and thermocouples are normalized to the same unit of measure (°C or °F).
- This ensures consistent analytics and reduces errors.
- Contextualization Through Metadata:
- Adding metadata tags (e.g., timestamp, sensor location) provides operational context.
- For instance, associating vibration patterns with past maintenance records enhances failure prediction accuracy.
4.1.4. Real-Time Monitoring and Anomaly Detection:
Continuous real-time monitoring is essential for identifying anomalies and potential threats in cPdM systems.
- Machine Learning for Anomaly Detection:
- Advanced ML algorithms analyze sensor data to detect deviations from normal patterns.
- For example:
- Unusual vibration levels may indicate bearing failure, triggering preventive maintenance.
- This proactive detection reduces unplanned downtime and mitigates security risks.
- Automated Alerts and Incident Response:
- Real-time alerts notify maintenance teams of potential issues.
- Automated incident response protocols ensure quick action to mitigate threats.
4.1.5. Edge Computing for Enhanced Security:
Edge computing enhances both data security and operational efficiency by processing data locally, reducing exposure to cyber threats.
- Local Data Processing:
- Instead of transmitting all data to the cloud, edge devices process critical data on-site.
- For example:
- Lubrication temperature data can be processed locally to detect potential overheating issues in rotating machinery.
- Lubrication temperature data can be processed locally to detect potential overheating issues in rotating machinery.
- Reduced Network Exposure:
- Minimizes the volume of sensitive data transmitted over the network.
- Lowers the risk of interception during data transfer.
- Faster Decision-Making:
- Reduces latency for real-time responses, enhancing operational efficiency.
4.2. Compliance with Regulatory Standards
To comply with industry regulations, cPdM systems must implement governance frameworks and conduct regular risk assessments. This ensures the security, accuracy, and transparency of data management practices.
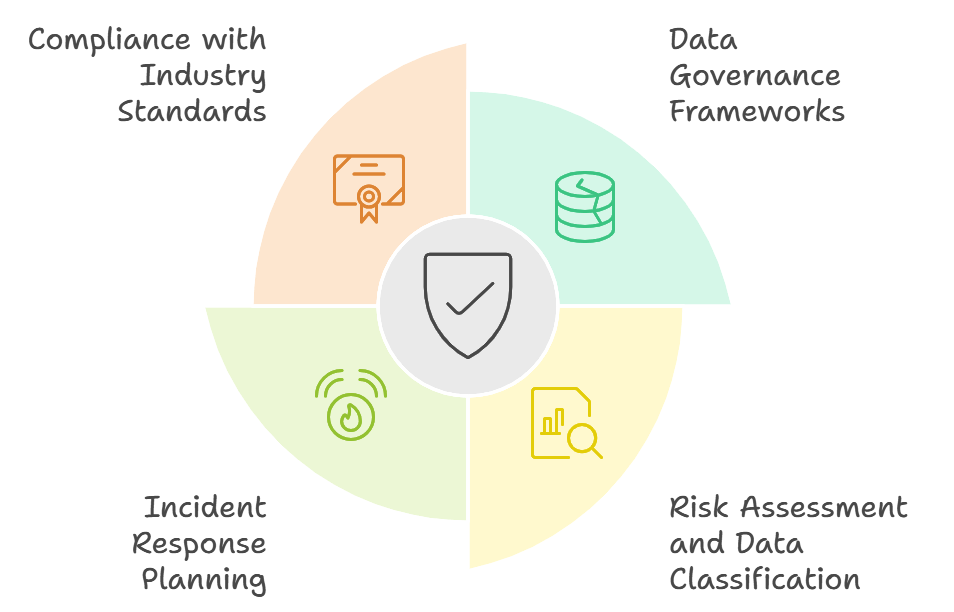
4.2.1. Data Governance Frameworks:
Establishing data governance frameworks is vital for ensuring regulatory compliance in cPdM.
- Policy Definition:
- Organizations define policies covering:
- Data retention periods.
- Access controls.
- Audit trails tracking who accessed what data and when.
- This enhances data transparency and facilitates regulatory audits.
- Organizations define policies covering:
- Compliance with Regulations:
- Adhering to GDPR, HIPAA, or CCPA ensures data privacy and regulatory compliance.
4.2.2. Risk Assessment and Data Classification:
Conducting regular risk assessments helps identify vulnerabilities and classify data based on sensitivity levels.
- Data Classification:
- Categorizing data as:
- Confidential: Maintenance logs and failure reports.
- Internal: Routine operational data.
- Tailoring security measures based on data sensitivity.
- Categorizing data as:
- Ongoing Risk Assessment:
- Regularly evaluating cybersecurity risks.
- Adjusting security protocols accordingly.
4.2.3. Incident Response Planning:
A robust incident response plan ensures that organizations can effectively handle security breaches in cPdM.
- Incident Response Team (IRT):
- A dedicated team trained to:
- Contain security incidents quickly.
- Minimize downtime and data loss.
- A dedicated team trained to:
- Post-Incident Reviews:
- Conducting reviews to identify root causes.
- Strengthening future response protocols.
4.2.4. Compliance with Industry Standards:
Following recognized industry standards ensures consistent security practices and compliance with regulations.
- ISO 27001 and NIST Frameworks:
- Guide organizations in data protection, risk management, and compliance.
- Regular audits verify adherence to these standards.
5.0. Conclusion

In today’s data-driven industrial landscape, data security and regulatory compliance play a vital role in the successful implementation of Continuous Predictive Maintenance (cPdM). Industries where operational integrity and adherence to regulations are paramount must prioritize secure data management to safeguard sensitive information and maintain trust with stakeholders.
By applying Industrial DataOps principles, organizations can build a robust security framework that not only protects data but also ensures full compliance with industry standards. This is achieved through:
- Encrypted data acquisition protocols that prevent unauthorized access during transmission.
- Role-Based Access Control (RBAC) to restrict data accessibility to authorized personnel only.
- Real-time anomaly detection powered by machine learning, enabling swift identification and response to potential security breaches.
Moreover, cPdM governance frameworks establish clear guidelines for data handling, retention, and access control, promoting transparency and regulatory adherence. Regular risk assessments and data classification strategies further strengthen security by allowing organizations to prioritize sensitive data protection.
By aligning with globally recognized standards such as ISO 27001 and the NIST Cybersecurity Framework, organizations can maintain high-security standards while continuously monitoring and improving their defense strategies.
As industries evolve toward more connected ecosystems, the importance of proactive data security and compliance will only increase. The seamless integration of Industrial DataOps into cPdM not only enhances operational efficiency but also fortifies cyber resilience, helping organizations maintain their competitive edge while safeguarding valuable industrial data.
6.0. Related Publications
- #015: Can your PM do this? - Part 1
- #016: Can your PM do this? - Part II
- #025: Can your PM really do this - Part III?
7.0. ContinuousTV Audio Podcasts
- AP006: Can your AI/ML Stack do this? Predict, Monitor and Retrieve.
- AP007: Is your PLC an MTP based on Margo?
- AP008: Industrial DataOps - Part 1
8.0. Latest AI News
- 𝗚𝗼𝗼𝗴𝗹𝗲: 𝟭𝟴𝟱 𝗴𝗮𝗺𝗲-𝗰𝗵𝗮𝗻𝗴𝗶𝗻𝗴 𝗔𝗜 𝘂𝘀𝗲 𝗰𝗮𝘀𝗲𝘀 𝘆𝗼𝘂 𝗰𝗮𝗻'𝘁 𝗮𝗳𝗳𝗼𝗿𝗱 𝘁𝗼 𝗺𝗶𝘀𝘀—𝗵𝗼𝘄'𝘀 𝘆𝗼𝘂𝗿 𝗶𝗻𝗱𝘂𝘀𝘁𝗿𝘆 𝘁𝗿𝗮𝗻𝘀𝗳𝗼𝗿𝗺𝗶𝗻𝗴?
- 🤖 𝗚𝗿𝗼𝘂𝗻𝗱𝗯𝗿𝗲𝗮𝗸𝗶𝗻𝗴 𝗡𝗲𝘄𝘀 𝗶𝗻 𝗔𝗜 & 𝗥𝗼𝗯𝗼𝘁𝗶𝗰𝘀: 𝗠𝗲𝘁𝗮'𝘀 𝗟𝗮𝘁𝗲𝘀𝘁 𝗜𝗻𝗻𝗼𝘃𝗮𝘁𝗶𝗼𝗻 𝗥𝗲𝘃𝗼𝗹𝘂𝘁𝗶𝗼𝗻!
9.0. FAQs
share this