cLCG for AI Apps: Ensuring Compliance & Ethical AI
Explore how Continuous Lifecycle Governance (cLCG) ensures ethical AI deployment & GxP compliance throughout the AI lifecycle. Learn key benefits & challenges.
share this

As artificial intelligence (AI) becomes increasingly integral to various industries, including life sciences and finance, the safe, ethical, and continuous validation of AI has emerged as a top priority. This blog explores why Continuous Lifecycle Governance (cLCG) is critical in maintaining compliance and ensuring the long-term success of AI products in highly regulated environments.
“You will need Continuous Governance built into any AI app that is deployed in the GxP arena. We have partnered with IBM to ensure all our AI models are continuously validated leveraging watsonx.gov. Our mantra is not just validation but continuous validation in everything we provide.
Nagesh Nama, CEO, xLM
1.0. Introduction: Why Continuous Lifecycle Governance (cLCG) for AI is Essential
AI is dynamic and ever-evolving, and this evolution introduces potential risks that require a governance model capable of adapting accordingly. In industries like life sciences and finance, which are governed by stringent regulations, the continuous lifecycle governance (cLCG) model is a foundational framework to ensure compliance and ethical decision-making.
1.1. What is Continuous Lifecycle Governance (cLCG) for AI?
Continuous Lifecycle Governance for AI refers to a set of protocols that govern the AI system from inception through deployment, continuous monitoring, and eventual retirement. This ensures that AI systems meet regulatory standards and ethical guidelines while addressing risks such as model drift, bias, and performance degradation.
2.0. Why cLCG is Essential for AI in Regulated Industries
AI is dynamic and ever-evolving from the moment an AI system is conceived to its deployment and ongoing use, its nature and impact change. This evolution introduces potential risks and necessitates a governance model that can adapt accordingly.

2.1. Ethical Concerns and Trust in AI Governance
AI decisions can have significant regulatory and ethical implications, particularly in sensitive sectors like GxP manufacturing. It is essential to ensure that AI models are fair, transparent, and accountable. This is particularly important for life sciences and finance, where AI decisions can directly affect human health, safety, and finances.
By embedding continuous governance, organizations ensure that their AI systems are consistently monitored and validated to prevent biases or unintended harm.
2.2. Regulatory Compliance: Ensuring Ongoing Adherence to Standards
With increasing global regulatory pressure, such as the EU’s AI Act and GDPR, it is critical to adapt AI systems in real-time to remain compliant. For GxP applications using AI, integrated continuous governance is the only viable option.
2.2.1. How does Continuous Lifecycle Governance ensure AI compliance in regulated industries?
By providing real-time checks and balances during every stage of the AI lifecycle, cLCG ensures that AI models comply with changing regulatory requirements and remain aligned with ethical standards.
2.3. Mitigation of Risks in AI Systems
AI models can experience model drift, which occurs when a model's performance degrades due to changes in data patterns or unforeseen anomalies. Continuous monitoring within the framework of cLCG helps mitigate these risks before they escalate into larger issues such as biased outputs or security vulnerabilities.
2.3.1. How does cLCG mitigate AI risks in life sciences and finance?
By continuously validating and updating AI models, cLCG ensures that any issues—such as data drift or algorithmic bias—are promptly identified and addressed, protecting stakeholders from harmful outcomes.
2.4. Maximizing the Value of AI Systems
AI systems are long-term investments that need ongoing optimization to provide lasting value. By implementing governance protocols such as cLCG, organizations can ensure that AI models remain relevant, effective, and aligned with market demands and regulatory needs.
3.0. How Continuous Lifecycle Governance (cLCG) Works for AI
Implementing cLCG involves integrating governance protocols at every phase of the AI lifecycle, ensuring continuous monitoring, compliance checks, and real-time updates.
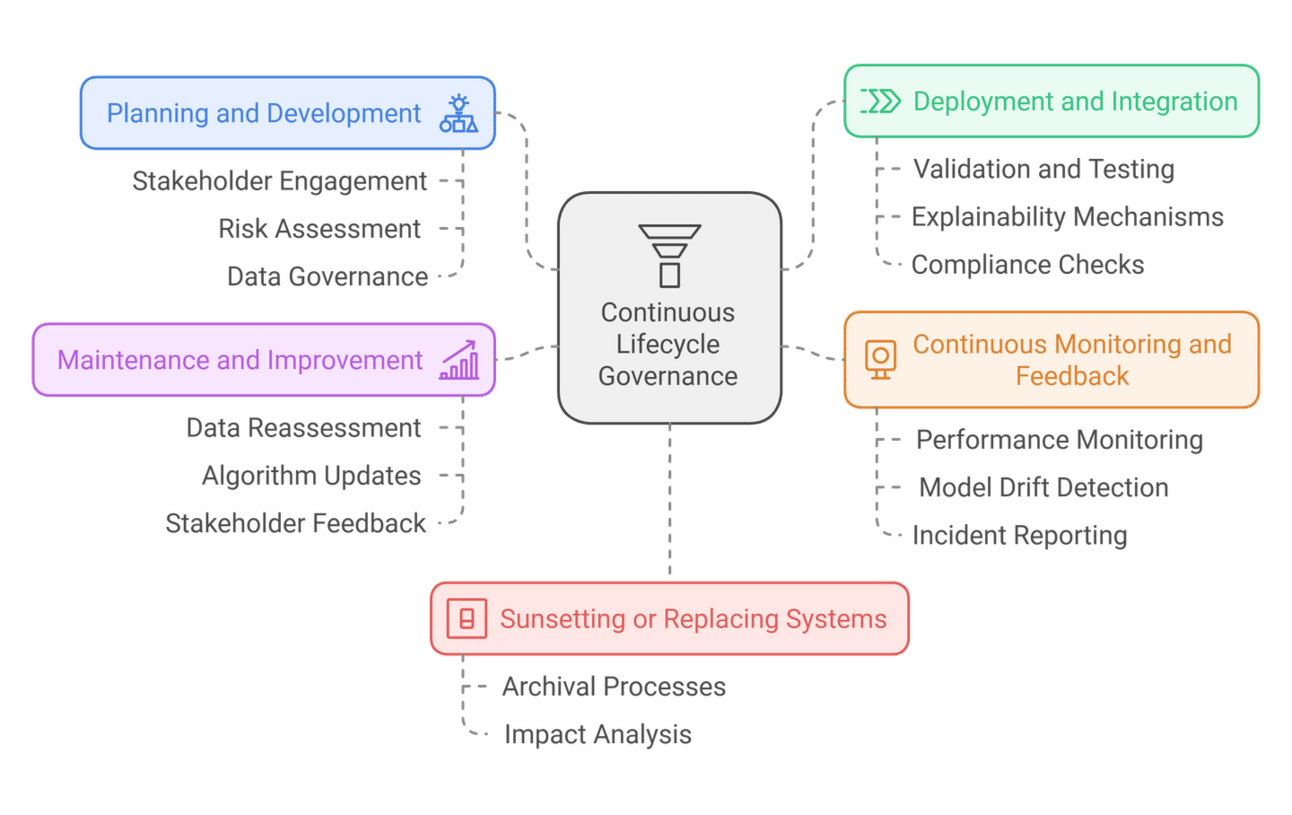
Here’s a detailed breakdown of its key components:
3.1. Planning and Development: Establishing a Strong Governance Foundation
The process begins by defining clear objectives and ethical guidelines. This includes:
- Stakeholder Engagement: Collaborating with diverse teams to align on goals and risks.
- Risk Assessment: Identifying potential biases, vulnerabilities, and societal impacts.
- Data Governance: Ensuring high-quality, unbiased, and representative datasets.
Governance at this stage lays a solid foundation for transparency and accountability in AI systems.
3.2. Deployment and Integration: Ensuring Operational Integrity
Once an AI model is deployed, governance protocols ensure it performs as expected, aligns with regulatory standards, and remains transparent.
- Validation and Testing: Conducting rigorous testing to verify that the model performs as intended across various scenarios on an ongoing basis.
- Explainability Mechanisms: Incorporating tools that make AI decision-making transparent for both technical and non-technical stakeholders.
- Compliance Checks: Ensuring that all regulatory and ethical requirements are met prior to deployment.
3.3. Continuous Monitoring and Feedback: Real-Time Governance
Even after deployment, cLCG doesn't stop. It intensifies:
- Performance Monitoring: Tracking metrics such as accuracy, fairness, and efficiency to ensure consistent performance on a continuous basis.
- Model Drift Detection: Identifying and addressing deviations in behavior due to evolving data patterns.
- Incident Reporting: Establishing a feedback loop to capture anomalies, user complaints, or ethical concerns.
3.4. Maintenance and Improvement
AI systems need periodic updates to stay effective and compliant.
- Data Reassessment: Ensuring datasets remain relevant and representative over time.
- Algorithm Updates: Implementing new techniques or insights to enhance model performance.
- Stakeholder Feedback: Regularly engaging stakeholders to refine governance policies based on real-world use.
3.5. Sunsetting or Replacing Systems: Ensuring a Responsible Transition
When an AI system becomes obsolete or is replaced, governance protocols ensure a responsible transition:
- Archival Processes: Safeguarding historical data for future reference or audits.
- Impact Analysis: Assessing the broader effects of retiring an AI system, including data dependencies and user disruptions.
4.0. Challenges in Implementing cLCG for AI
While cLCG offers numerous benefits, its effective implementation requires overcoming several challenges:
- Resource Intensity: Continuous monitoring and updates demand dedicated resources and skilled personnel. xLM’s guardrails for GxP provides a validated solution.
- Interdisciplinary Coordination: Effective governance necessitates collaboration among data scientists, legal/regulatory experts, ethicists, and business leaders.
- Technological Complexity: Ensuring explainability and interpretability in complex models, such as neural networks, can be daunting. xLM’s data scientists build, test, and validate highly complex ML models.
- Scalability: As organizations deploy more AI systems, scaling governance frameworks without compromising effectiveness becomes critical. xLM has partnered with IBM to deliver cLCG that is infinitely scalable.
However, xLM’s partnership with IBM ensures that cLCG can scale infinitely without compromising effectiveness.
5.0. Conclusion: Paving the Path for Continuously Validated AI
Continuous Lifecycle Governance (cLCG) is more than just a set of policies—it's a mindset that prioritizes responsibility, adaptability, and long-term value for AI systems. By embedding governance into every phase of the AI lifecycle, organizations can create resilient, trustworthy AI systems that align with regulatory and ethical standards.
5.1. Why is continuous validation for GxP compliance in AI apps essential?
For regulated industries, embedding continuous governance into AI apps ensures that models meet ever-evolving regulations, reduce risks, and maximize their long-term value.
Organizations embracing cLCG will not only mitigate risks but also position themselves as leaders in the responsible and sustainable deployment of AI in regulated industries.
6.0. ContinuousTV Audio Podcasts
- AP011: Can your CDMS to this?
- AP012: Are you a Biotech or a Medtech? Here are the 8 Out of the Box Apps that can run your company….
7.0. Latest AI News
share this