How AI Transforms CDMS: Smarter Clinical Data Management
Discover how AI-driven CDMS enhances clinical data accuracy, boosts efficiency, ensures compliance, and reduces manual errors for faster, smarter trials.
share this
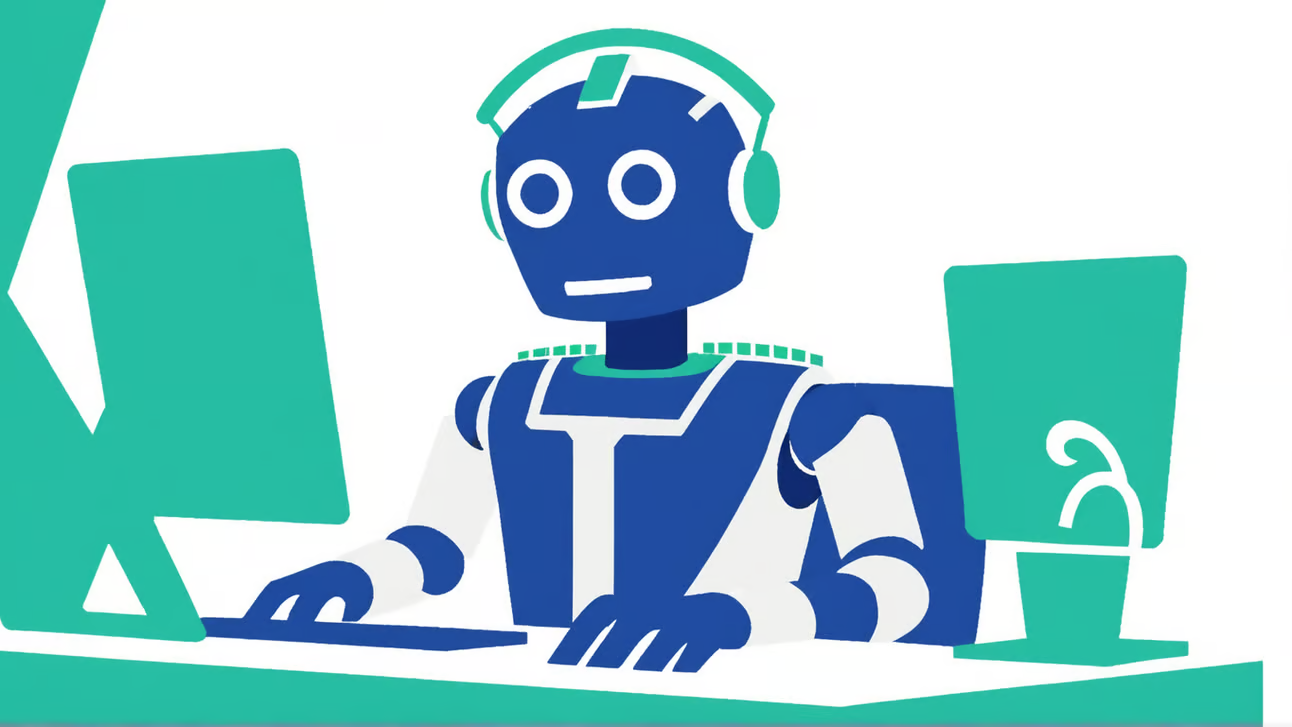
1.0. Introduction to Traditional Clinical Data Management Systems (CDMS)
Clinical Data Management Systems (CDMS) have been a cornerstone of clinical research for decades, ensuring that trial data is accurate, secure, and compliant with regulatory standards. However, traditional CDMS platforms come with several limitations that impact efficiency and scalability.
1.1. Key Features of Traditional CDMS:
- Data Collection – Information is manually entered from clinical sites, labs, and electronic health records (EHRs). This process is prone to transcription errors and delays.
- Data Validation – Ensures compliance with regulatory requirements through manual checks and automated validation rules. However, this step is often time-consuming.
- Data Analysis & Reporting – Statistical tools are used to generate reports for stakeholders, but manual intervention increases processing time.
- Regulatory Compliance – Adherence to Good Clinical Practice (GCP) and other guidelines is mandatory, adding layers of complexity to data management.
1.2. Challenges of Traditional CDMS:
- Manual Processes – Increased risk of human error, slowing down data processing.
- Scalability Issues – Struggles to handle large volumes of data in modern clinical trials.
- Limited Automation – Relies on human oversight, making trial management less efficient.
- Compliance Risks – Requires continuous monitoring to meet evolving regulatory standards.
As clinical trials become more complex, the need for AI-driven automation in CDMS is growing. Advanced solutions can enhance data accuracy, efficiency, and compliance, reducing the burden on clinical research teams.
2.0. How Traditional CDMS Platforms Work
Traditional Clinical Data Management Systems (CDMS) play a vital role in clinical trials by ensuring accurate data collection, validation, analysis, and reporting. However, these legacy systems often depend on manual processes, leading to inefficiencies and errors.
Below is a breakdown of how traditional CDMS platforms function and the challenges they face in modern clinical research.
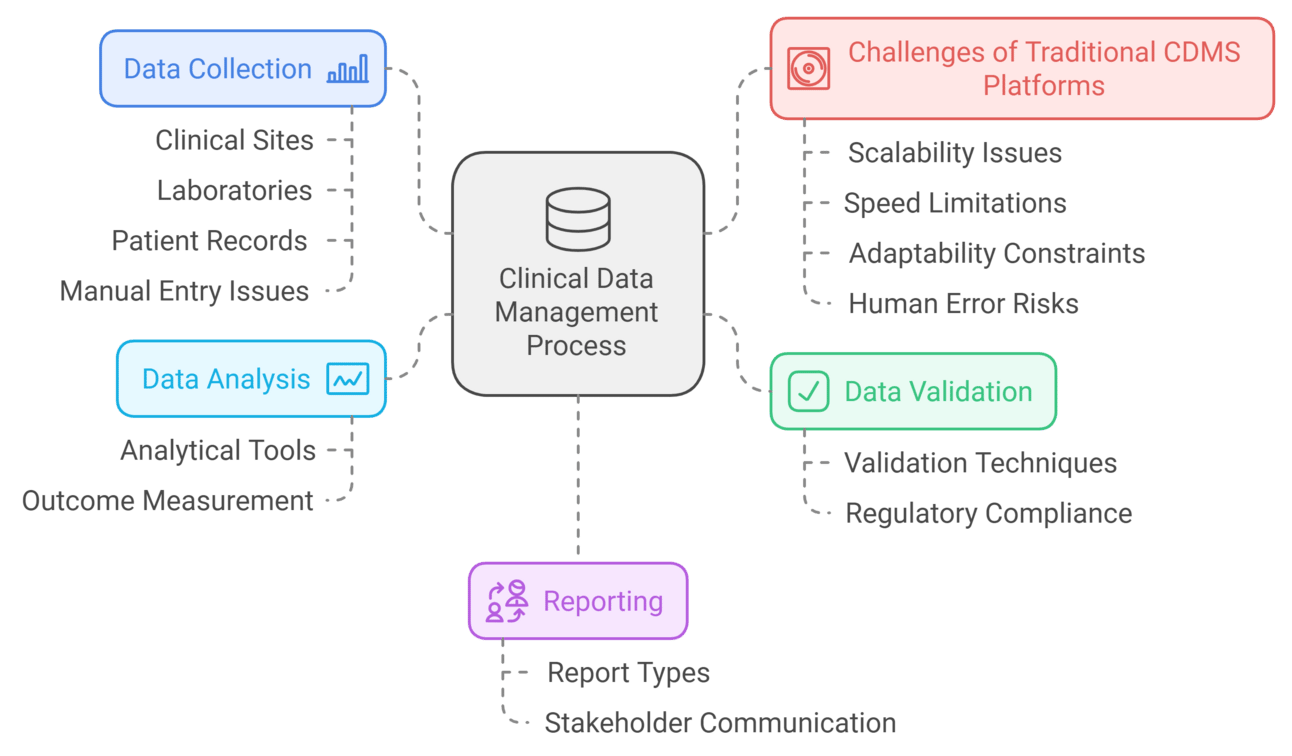
2.1. Data Collection
Data is gathered from various sources such as clinical sites, laboratories, and patient records. This data is often entered manually into the system, which can lead to inconsistencies and errors if not carefully monitored. The reliance on manual entry increases the time required for data collection and poses risks related to data integrity.
- Key Data Sources in Traditional CDMS:
- Clinical Sites: Collect patient-reported data and trial observations.
- Laboratories: Provide test results and biomarker data.
- Electronic Health Records (EHRs): Store patient history and medical background.
- Challenges of Manual Data Entry:
- Risk of Transcription Errors: Human errors compromise data accuracy.
- Time-Consuming Processes: Manual entry leads to data submission delays.
- Lack of Real-Time Access: Slower updates hinder decision-making.
2.2. Data Validation
Once collected, rigorous data validation checks are performed to ensure data integrity and compliance with regulatory requirements. This step often requires significant human oversight, which can increase the time needed for data readiness. Validation processes include range checks, consistency checks, and format checks to confirm that the clinical trial data meets predefined standards.
- Validation Techniques:
- Double Data Entry: Minimizes transcription errors and enhances accuracy.
- Automated Validation Rules: Detects inconsistencies, missing values, and incorrect formats.
- Statistical Checks: Identifies data anomalies and trends for better quality control.
- Regulatory Compliance:
- Adhering to Good Clinical Practice (GCP) ensures data accuracy and reliability.
- Following FDA and EMA guidelines helps maintain compliance in clinical trials.
Ensuring high-quality data validation within a Clinical Data Management System (CDMS) improves data accuracy, regulatory compliance, and overall trial efficiency.
2.3. Data Analysis
After data validation, the next critical phase in a Clinical Data Management System (CDMS) is data analysis, where validated data is examined to extract meaningful insights that drive clinical trial decisions. This process involves complex statistical analysis and customized reporting methods designed to meet the specific needs of stakeholders such as researchers, sponsors, and regulatory bodies.
- Analytical Tools:
- SAS and R: Widely used statistical software for clinical trial data analysis.
- Machine Learning & AI-driven Analytics – Enhances pattern recognition and predictive modeling.
- Outcome Measurement:
- Key Performance Indicators (KPIs): Evaluates trial success, patient outcomes, and data reliability.
- Data Visualization Techniques: Graphs and reports help in interpreting clinical trial results effectively.
A robust data analysis framework ensures that clinical trial data is accurate, insightful, and compliant with regulatory standards, ultimately leading to better decision-making in clinical research.
2.4. Reporting
The final step in Clinical Data Management Systems (CDMS) is reporting, where analyzed data is compiled into structured clinical trial reports for stakeholders. These reports must strictly follow Good Clinical Practice (GCP) guidelines to ensure accuracy, transparency, and compliance with regulatory authorities.
- Report Types:
- Interim Analysis Reports: Provides ongoing insights into clinical trial progress.
- Final Study Reports: Summarizes the complete trial findings for submission.
- Regulatory Submission Documents: Ensures compliance with FDA, EMA, and other regulatory agencies.
- Stakeholder Communication:
- Effective data reporting enhances communication between researchers, sponsors, regulatory bodies, and ethics committees.
- Well-structured reports ensure clarity, consistency, and regulatory acceptance.
While traditional CDMS platforms have effectively supported clinical research over the years, they face challenges in scalability, speed, and adaptability to changing requirements.
2.5. Challenges of Traditional CDMS Platforms
Traditional Clinical Data Management Systems (CDMS) have long been the backbone of clinical trials, but as the industry evolves, these legacy systems face significant challenges that impact efficiency, scalability, and compliance.
2.5.1. Key Limitations of Traditional CDMS:
- Scalability Issues: As clinical trials expand in size and complexity, traditional CDMS platforms struggle to handle large volumes of clinical data efficiently.
- Speed Limitations: Manual data entry and validation slow down workflows, leading to delays in data analysis and critical decision-making.
- Adaptability Constraints: Evolving regulatory requirements and trial protocol changes often require extensive system modifications, making traditional CDMS less flexible.
- Human Error Risks: A high reliance on manual data management increases the likelihood of errors, jeopardizing data integrity and compliance.
These challenges highlight the need for innovation in clinical data management, paving the way for AI-driven CDMS solutions that enhance efficiency, accuracy, and compliance..
3.0. CDMS Platforms Using AI Agents: Transforming Clinical Data Management
The integration of Artificial Intelligence (AI) in Clinical Data Management Systems (CDMS) is revolutionizing traditional data management processes. By leveraging AI-driven automation, organizations can significantly enhance data accuracy, efficiency, and regulatory compliance in clinical trials.

The AI agent can perform several key tasks:
3.1. Natural Language Processing (NLP)
The AI model ingests script requirements in natural language and converts them into executable CDMS scripts. This involves advanced NLP techniques that allow the system to understand user intent and generate corresponding code accurately.
- Applications of NLP: NLP can streamline communication between researchers and systems by allowing users to input requests in everyday language.
3.2. Automated Script Loading
The agent loads these scripts into the CDMS Integrated Development Environment (IDE) without manual input. This automation minimizes errors associated with manual loading and ensures scripts are ready for execution promptly.
- Efficiency Gains: Automated script loading reduces setup time for new studies or changes in protocols.
3.3. Navigation Path Optimization
The AI agent automatically determines the navigation path necessary to activate scripts within the CDMS interface. By analyzing user interface elements and workflow patterns, it identifies the most efficient route, saving time during script execution.
- User Experience Improvement: Optimized navigation paths enhance user satisfaction by reducing frustration associated with complex interfaces.
3.4. Workflow Grouping
To enhance organization and accessibility, the agent groups scripts by workflow type. This categorization allows users to easily locate relevant scripts based on their specific tasks or projects, improving overall workflow management.
- Task Management: Grouping workflows facilitates better project management by allowing teams to prioritize tasks effectively.
3.5. Workflow Execution
Once scripts are loaded and organized, the agent executes workflows seamlessly. It ensures that all necessary steps are performed in sequence without manual oversight, which is crucial for maintaining data integrity during clinical trials.
- Consistency Assurance: Automated execution reduces variability in how workflows are performed across different studies or teams.
3.6. GxP Compliant Documentation
After executing workflows, the agent generates a PDF document containing results formatted according to Good Practice (GxP) standards. This documentation is vital for regulatory compliance and provides stakeholders with clear insights into trial outcomes.
- Regulatory Readiness: GxP-compliant documentation simplifies audits by providing clear records of all processes followed during a trial.
3.7. Continuous Learning from Feedback
The AI model is designed to take feedback from users regarding script performance and execution results. By implementing reinforced learning techniques, it can adapt over time, enhancing its accuracy and efficiency based on real-world usage patterns.
- Adaptive Learning: Continuous improvement mechanisms allow AI agents to refine their processes based on user interactions over time.
3.8. Data Scrubbing for Privacy Compliance
Ensuring sensitive information is handled appropriately is paramount; therefore, the system includes functionality for data scrubbing—removing client-specific data from datasets before analysis or reporting to ensure compliance with privacy regulations such as GDPR or HIPAA.
- Privacy Protection Measures: Data scrubbing not only ensures compliance but also builds trust with participants regarding their personal information security.
3.9. Integration with Existing Systems
The AI agent can be integrated with existing clinical systems and databases to facilitate seamless data flow across platforms. This interoperability enhances collaboration among different teams involved in clinical trials.
- Cross-System Collaboration: Integration fosters a cohesive environment where different departments can share insights more effectively.
3.10. User-Friendly Interface
AI-driven CDMS platforms can feature an intuitive user interface that simplifies interactions for non-technical users. By providing visual cues and guided workflows, it helps users navigate complex processes more easily.
- Training Reduction: A user-friendly interface minimizes training time required for new users entering the system.
4.0. Advantages of Using AI Agents for CDMS Platform Design
Integrating AI agents into Clinical Data Management Systems (CDMS) revolutionizes data management by automating processes, improving accuracy, and ensuring compliance. These intelligent systems offer significant advantages that enhance clinical trial efficiency and data integrity.
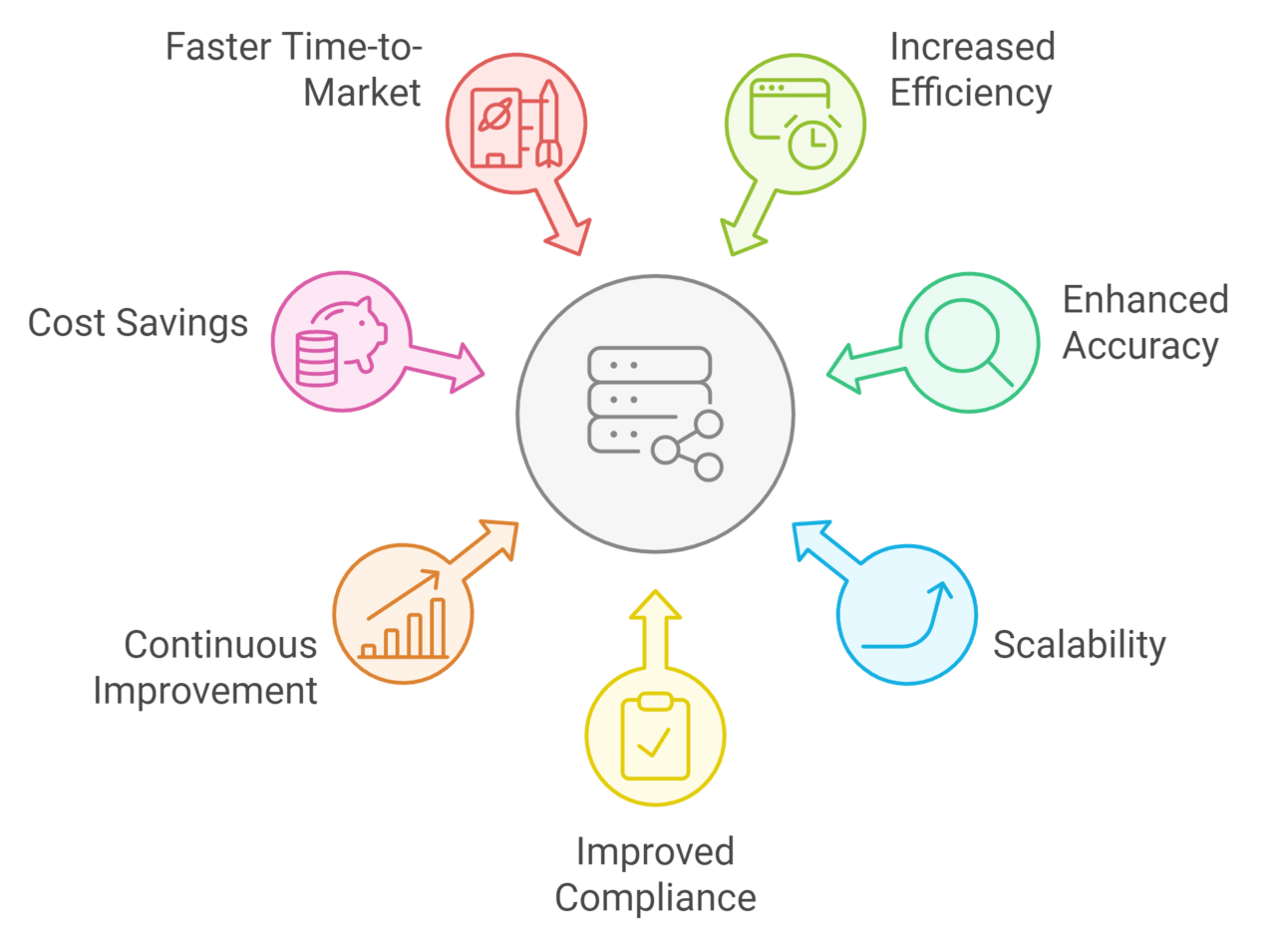
4.1. Key Benefits of AI Agents in CDMS Platform Design
- Increased Efficiency: AI-driven automation reduces manual tasks such as data entry, validation, and processing, allowing researchers to focus on critical analysis.
- Enhanced Accuracy: Advanced AI algorithms minimize human errors in data handling, ensuring more reliable clinical trial outcomes.
- Scalability: AI agents seamlessly adapt to growing data volumes, evolving protocols, and complex workflows, eliminating the need for extensive resource allocation.
- Regulatory Compliance: Automated documentation aligns with industry standards, such as GxP, HIPAA, and GDPR, reducing the risk of non-compliance in clinical trials.
- Continuous Learning & Improvement: AI systems leverage machine learning to analyze feedback and refine their performance over time, leading to more efficient data management.
- Cost Reduction: Automating repetitive tasks cuts operational costs, making clinical trials more budget-friendly while maintaining high-quality data management.
- Faster Time-to-Market: Optimized processes speed up data analysis, expediting decision-making and accelerating drug development timelines.
By leveraging AI-powered CDMS platforms, clinical research organizations can enhance efficiency, reduce errors, and maintain compliance while driving innovation in drug development.
5.0. Conclusion
The transition from traditional Clinical Data Management Systems (CDMS) to AI-driven CDMS platforms marks a transformative shift in clinical research. By automating critical processes, enhancing accuracy, and ensuring regulatory compliance, AI-powered CDMS solutions are set to revolutionize clinical trial management.
While traditional CDMS platforms have provided a solid foundation, integrating AI technology significantly boosts operational efficiency, data integrity, and compliance with GxP, HIPAA, and GDPR standards. These advancements not only streamline clinical data management but also contribute to faster drug development and improved patient outcomes.
As AI in clinical research continues to evolve, the synergy between advanced automation tools and human expertise will redefine industry standards. AI-driven CDMS platforms empower researchers to focus on what truly matters—enhancing healthcare, accelerating clinical trials, and advancing medical innovation on a global scale.
Note: Such an AI driven system shall be managed under a Continuous Governance framework that ensures the outputs are predictable with no exceptions. Such a Governance Framework incorporates Continuous Validation.

6.0. ContinuousTV Audio Podcasts
- AP008: Industrial DataOps - Part 1
- AP009: Industrial DataOps - Part 2
- AP010: Industrial DataOps Part 3 - DataOps + Continuous Predictive Maintenance (cPdM)
7.0. Latest AI News
- 𝘾𝙖𝙣 𝘼𝙄 𝙨𝙖𝙫𝙚 𝙡𝙞𝙫𝙚𝙨 𝙗𝙚𝙛𝙤𝙧𝙚 𝙗𝙞𝙧𝙩𝙝?
- AI is set to revolutionize the fight against a parasitic disease that's been neglected for too long—here's how
- Are you ready for AI to rewrite the rules of reality by 2026?
8.0. FAQs
share this